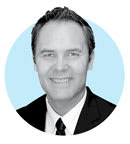
FEELING OVERWORKED and dragged down by an ever-increasing to-do list? With technology and innovation, this was not what was planned.
Nearly a century ago, economist John Maynard Keynes proposed that by the middle of the 20th century, we would be working only 15 hours a week (Keynes, 1930). The 1960s and 1970s were seen as a time when the process might evolve to a shorter workweek and more leisure time, but the opposite occurred: by 2005, Americans were working an average of five more weeks per year compared to the 1970s (Mastny and Hunnicutt, 2013).
Expecting better innovation and technology would create more leisure time proved to be misguided. Nevertheless, machines and artificial intelligence (AI) have made advancements in our lives, and as these innovations progress, the goal and aim in health care is better care for patients.
Ultimately, this evolution will infuse all aspects of our treatment of patients, from medication selection to surgery. But before AI is able to recommend the best treatments, we must continue to innovate in the area of diagnostics.
Diagnostics and management of conditions require the evaluation of data comparing patients’ health to a norm. Without knowing what a normal corneal shape is, one would not know that a keratoconus cornea was irregular. Likewise, without understanding the appearance of a normal retina, one would not be able to teach AI to discover and mark diabetic retinopathy as abnormal.
An excellent way to utilize AI is to upload images to it to develop a normative database (assuming one is following all laws and regulations in disclosing images, particularly to open-internet AI systems). When equipment is purchased from manufacturers, a database is usually embedded in the software to compare with patients’ images.
For example, if an Inferior/Superior (IS) difference metric on topography is particularly large, AI embedded within the topographer will signal that somehow this is askew. Currently, retinal cameras are being used outside the practice of eye care to image patients’ retinas and signal whether a patient may need an additional retinal evaluation because of suspect diabetic retinopathy.
The concept of data from one software system working alongside software from a different system is termed interoperability. As interoperability improves, it becomes possible to upload images from various instruments and have AI evaluate aspects of patients’ topographies from both machines. This will allow for a huge database that includes both normative and abnormal data. While this technology is already in the works, especially in large institutions, strides must be made for complete synergy.
Custom contact lens laboratories are already taking advantage of this software by merging data from around the world. For instance, when a laboratory can accept data files of patients’ topographies, they can be merged with data from thousands of eyes within a database to better suggest and manufacture lenses.
If you do not want to be a laggard with this technology, call your laboratory and ask the technicians about how their consultants use data-driven systems in the creation of lenses. Some of the best laboratories are already using data-driven lens designs for nearly all their products. Once the products arrive in the office, the expertise and specialty of the fitter can assure that the lenses fit and work as planned.
While eyecare providers (ECPs) likely will never be sitting around trying to figure out what to do with their time, embracing AI may make ECPs’ examinations and diagnoses easier, more precise, and healthier for patients.
REFERENCES
1. Keynes JM. Economic Possibilities for our Grandchildren. 1930. Available at econ.yale.edu/smith/econ116a/keynes1.pdf. Accessed 2024 Feb 23.
2. Mastny L, Hunnicutt BK. Free Time: The Forgotten American Dream. 2013 June. Available at resilience.org/stories/2013-06-21/free-time-the-forgotten-american-dream. Resilience, originally published by Center for a New American Dream. Accessed 2024 Feb 23.