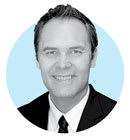
THERE HAS BEEN a tidal wave of innovation in the dry eye space over the last two decades. As far as I can see, more clinical changes have been implemented for the treatment and management of dry eye than for any other condition in the last 20 years. It appears, though, that dry eye frustrates artificial intelligence (AI) more than any other condition.
According to the Tear Film & Ocular Surface Society Dry Eye Workshop II (TFOS DEWS II) report, “Dry eye is a multifactorial disease of the ocular surface characterized by a loss of homeostasis of the tear film, and accompanied by ocular symptoms, in which tear film instability and hyperosmolarity, ocular surface inflammation and damage, and neurosensory abnormalities play etiological roles” (Craig et al, 2017).
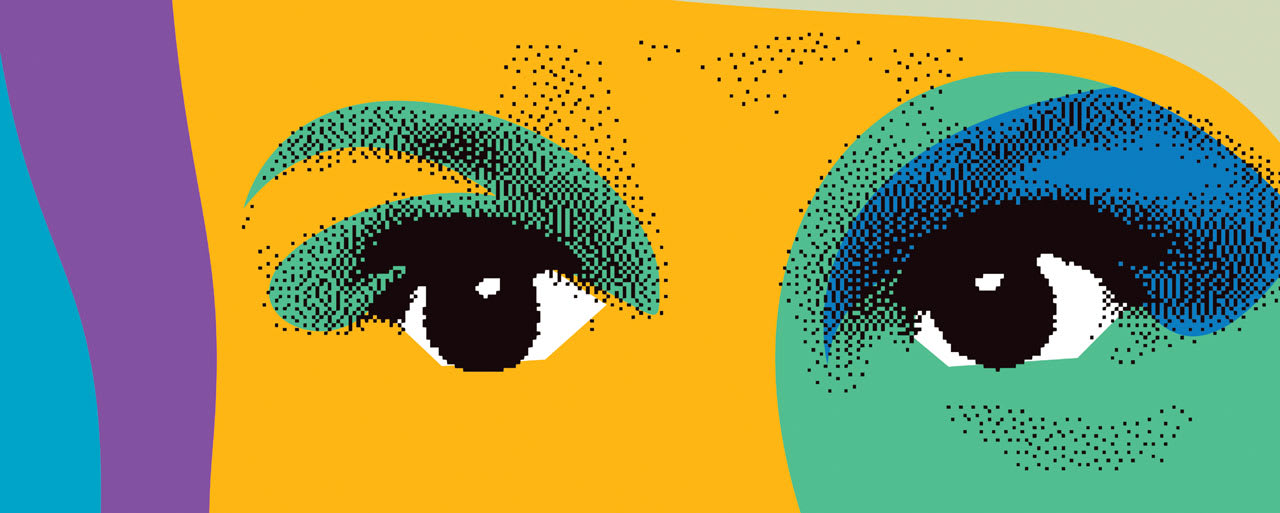
When artificial intelligence software was asked “What is AI good at when it comes to diagnosis and management?” it returned the following responses (Open AI, 2024):
• AI in health care excels at analyzing vast medical datasets to recognize patterns for accurate diagnosis, aiding clinicians in decision-making and enabling personalized treatment plans.
• AI interprets medical imaging data, expedites drug discovery, and facilitates remote monitoring.
• Overall, AI revolutionizes health care by enhancing diagnostic accuracy, accelerating research, and improving patient care delivery.
The problem, though, is that there are no vast clinical medical datasets, so we do not have good methods of measuring accurate diagnoses that have universal consensus. Medical imaging is not yet able to differentiate different types of dry eye. There seems to be too much variability in patient assessment, resulting in discrepancies in the evaluation and treatment of patients.
But hope remains. Here are some suggestions regarding what needs to happen:
• Streamline the process of measuring the chemical analysis of the tear film
• Better clarify (clinically and for artificial intelligence) of what homeostasis is
• Improve quantification of tear film instability through instrumentation
• Make osmolarity testing universal
• Quantify inflammation and surface damage and universally define and measure the neurosensory components of dry eye
These diagnostics can then be entered into a database to put myriad treatments at the eyecare professional’s disposal, then these measurements need to be recorded again.
While eyecare professionals can upload large databases of clinic research into AI programs, it would be challenging to know how multiple treatments might be combined to ensure the best outcomes for patients.
While this may not be the most optimistic viewpoint regarding the benefits that AI can bring to the exam process, to make a solid impact on our patients’ lives, we need to do more work in the dry eye space to advance our own clinical decision-making—then perhaps AI can be given the reins to make treatment recommendations for us.
REFERENCES
1. Craig JP, Nichols KK, Akpek EK, et al. TFOS DEWS II Definition and Classification Report. Ocul Surf. 2017 Jul;15:276-283.
2. "How AI Can Help in Medical Diagnosis and Treatments." In GPT-3.5 (Version 2) [Response to user query]. 2024.