PRACTITIONERS have been using artificial intelligence (AI) for decades as part of their practices. However, many didn’t realize what AI was until the last couple of years. Some may still think of it as an internet program with which we can ask fun questions, make funny pictures, and nothing more. Others are looking at the processing of data and realizing that the internet can multiply our capabilities and take us to new levels, similar to how baseline computing did in its early days.
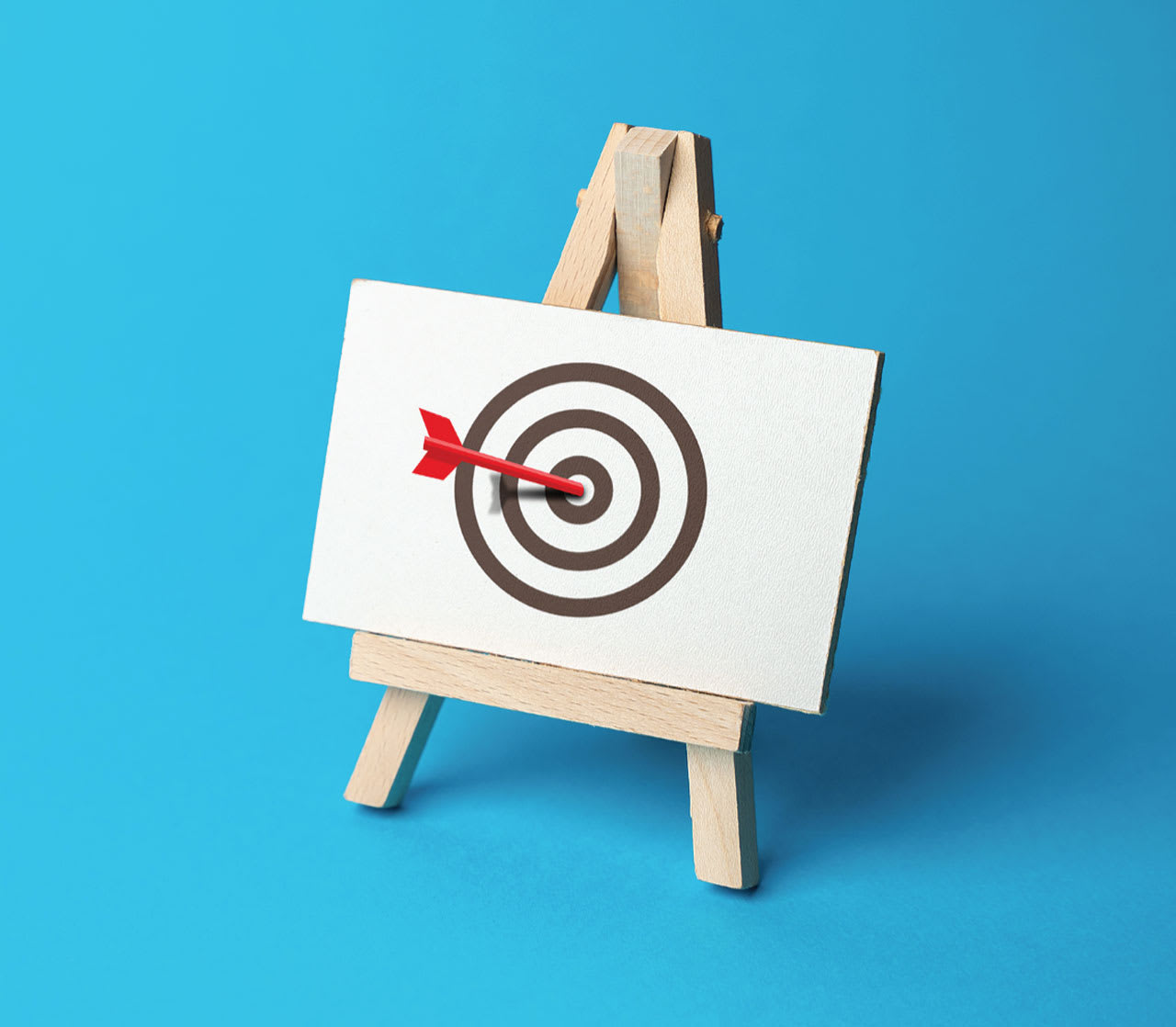
This column has previously covered the impact that large-scale databases can have on advancing contact lens-fitting capabilities. This month, let’s look at some examples of how this is being currently being done.
During my residency in 2004 and 2005, GP lenses for keratoconus were fitted using what is now referred to as a “traditional approach.” Based on the fitting guide for a specific lens, practitioners would select a posterior base curve lens using various methods. For some, the steep keratometry reading along with a correction factor was used to choose the initial lens, while other manufacturers directed providers to focus on the apex of the cone. Regardless of the method, the correct lens was usually found after about 5 or 6 trial lenses. This method became streamlined over time as an initial trial lens was selected by a more sophisticated method that was far less scientific (ie, starting with the middle of the fitting set).
One study looked at nearly 200 eyes to compare 17 different frameworks to a more traditional method of coming up with the ideal GP lens fit (Abadou et al, 2025). These frameworks were taught and could successfully predict the posterior radius of curvature of the best-fitting lens with various degrees. One method of topography evaluation that stood out used a convolutional neural network (CNN). This type of AI model is made for processing visual and specific data, in this case, topography images. This method, particularly, outperformed the process of using mean K and the specific lens-fitting guide.
Another study looked at a machine learning algorithm for orthokeratology (Zhou et al, 2024). This retrospective study evaluated nearly 800 eyes that had been previously fit and had good lens centration on topography. In addition, to refine things, the AI model used spherical refraction, keratometry reading, and corneal eccentricity, among other criteria. This AI-assisted method showed a higher rate of first-fit success with contact lenses.
Both of these studies employ a complicated method of using large databases to prove what practitioners may already know: When a large collection of data is put together to look at a new method of doing something, incredible trends come from it that provide for better clinical outcomes.
A revolution in the industry by our contact lens manufacturers is imminent. Some industry partners are already coming forward with algorithms that extrapolate the data from their databases and improve success. Some practitioners are already using them in clinic. For example, if you do empirical fitting and achieve first-fit success with a high degree of accuracy, you can be assured that the lab is already doing something of this sort using AI. With time, practitioners will see this become more mainstream and the degree of accuracy of first-fit empirical fitting will go way up!
References
1. Abadou J, Dahan S, Knoeri J, Leveziel L, Bouheraoua N, Borderie VM. Artificial intelligence versus conventional methods for RGP lens fitting in keratoconus. Cont Lens Anterior Eye. 2025;48:102321. https://doi.org/10.1016/j.clae.2024.102321
2. Zhou, X., Zeng, L., Shen, Y., Kang, P., Zhou, X., & Chen, Z. Artificial intelligence-assisted fitting method using corneal topography outcomes enhances success rate in orthokeratology lens fitting. Cont Lens Anterior Eye. 2024 Dec 16:102350. [Online ahead of print] doi.org/10.1016/j.clae.2024.102350